Research Idea
The identification and differentiation of tissues forms the basis of surgical therapy. Intraoperative tissue differentiation, together with imaging procedures and histopathological and laboratory chemical diagnostics, is one of the various scenarios that influence the decision on therapy. In addition to improved diagnostics, the minimization of surgical trauma during complete tumor removal (R0 resection) remains an essential goal of the further development of surgical methods. In oncology in particular, with its claim to complete tumour resection, new medical technology methods make it possible to clearly indentify tumor tissue and its specific differentiation from healthy tissue in all spatial dimensions minimizing damage to surrounding tissue while maintaining functional tissue structures. A reliable differentiation between healthy and malignant tissue is provided by the histopathological examination of the resected tissue, which also guides intraoperative decision-making in the context of frozen section diagnostics and represents the current state of the art. At the same time there is potential to reduce the time required, the quality and the tissue damage.
Research Focus A: Sensor Development
The development of sensor technologies is a central task of the research training group and represents the focus of the projects A1-A5. The individual sensors use the changes in optical, mechanical or electrical properties to distinguish between malignant and benign tissue.
Various studies show that biological tissues exhibit frequency-dependent differences in impedance and permittivity. A distinction between healthy and tumorous tissue is possible due to the changed physiology (stiffness, water and salinity, molecular composition) and the resulting changes in dielectric properties. It has already been shown that tumor tissue is characterized by greater capacitive properties and increased permittivity. In the case of breast cancer, approaches already exist for the classification of healthy and malignant tissue using neural networks or linear discriminant analysis, in which the Cole-Cole parameters or selected features in the impedance spectrum are considered. In the case of colorectal cancer, even a differentiation of the different tumor stages has been shown based on measurements of relative permittivity. In the PhD thesis the differentiation of healthy and malignant tissue based on impedance spectroscopy will be investigated and further developed. In contrast to the detection of electrical properties to support imaging procedures in preoperative diagnostics, this project aims to develop procedures for intraoperative applications. Bladder tumor resection serves as an exemplary procedure.
Research Focus B: Modelling and Classification
In order to be able to use the multimodal sensor data obtained in projects A1-A5 intraoperatively, a close link between the projects in priority area B is planned. The intraoperative information gain is embedded in a superordinate pre- and postoperative learning loop, which is intended to continuously improve the reliability statements. It is essential to validate the intraoperative results using the histopathological rapid section. In addition to the multimodal sensors from the projects A1-A5, the existing intraoperative camera image is used for dynamic image-based positioning, which supports the surgeon in aligning the instruments. The data-driven multimodal sensor fusion will not only receive the pure sensor signals, but also associated location information and pre-processed tissue parameters.
A meaningful fusion of the collected multisensory measurement data for classification requires a subordinate solution of some technical problems. Where are the recorded measuring points located? What are the connections in the tissue? How can the collected data be fused and classified? How can learning be done across different patients? For this purpose, a cross-domain modelling of tissue and sensor properties is realised and represents the interface between the focal areas "sensor technology" and "classification", in which the sensor information is merged. As a basis for tissue differentiation it is assumed that different tissue states (e.g. benign and malignant) have different properties, which in turn can be quantified by domain-specific tissue parameters. There are causal relationships between abnormal cell metabolism and the optical absorption spectra or between changes in the cell structure and the elasticity or compression behaviour. As a consequence, a selection of relevant tissue parameters can be derived and measured with the help of the sensor technology projects in the Research Training Group, which form the basis for differentiation. In order to use the information content of several sensor channels, the pure sensor signal must be provided with consistent local information.
The aim of this project is to use multivariate statistical methods to analyze the extensive data sets resulting from the different measurement procedures and to optimize the classification quality of the multimodal tissue differentiation procedures by taking local tissue parameters into account.
Research Focus C: Surgery and Pathology
In cooperation with the University Hospitals of Tübingen, tissue samples are made available to doctoral students. In addition, doctors are working on bladder tumour models, the validation of spectroscopic tissue differentiation methods and multiparametric tissue classifiers as part of the C1-C3 projects.
Project Partners
The project is funded by the DFG.
Bearbeiter
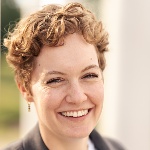
Carina Veil
Dr.-Ing.Head of the Medical Engineering Group
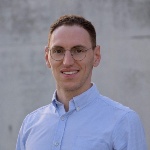
Matthias Ege
M.Sc.Research Assistant
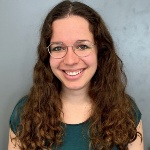
Franziska Krauß
M.Sc.Research Assistant